By John McHenry, Baron Chief Scientist
Providing decision support for a wide range of weather-sensitive societal and economic sectors is a vital part of the critical weather intelligence that Baron offers customers. The technology and ability to address real-time, nowcast, and forecast scale problems using multi-scale, multi-model, multi-method decision support systems are crucial to mitigating economic and personal safety threats, a core component of Baron’s global mission. This overview article considers the wide variety of forecast technologies broadly classified under decision support and documents representative use cases in which operational Baron DSS systems have provided actionable guidance.
Before discussing the technology, it is worthwhile to note that none of Baron’s DSS forecast technology is considered “off-the-shelf.” Baron takes a customer-oriented approach, first determining the customer’s needs, requirements, and desires. It then builds a solution around the customer’s context: what they need to utilize and disseminate the critical weather intelligence derived from a Baron DSS. While Baron has over 100 person-years of experience in developing and deploying model-based DSS forecast systems, the company is accustomed to working with customer-preferred models as well. This is often the best approach because many customers already have knowledge and experience around their local modeling systems but may not have the ability or resources to integrate them into the operational DSS, which is lacking. Baron thus brings to the table the eagerness to both “work-in-context” and to provide its own highly sophisticated modeling solutions when and where needed.
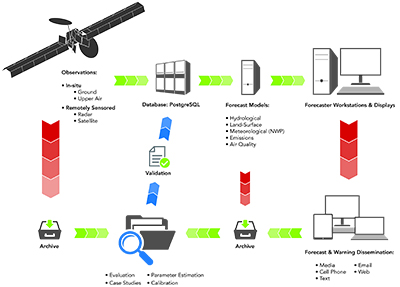
Figure 1. provides an overview of a conceptual DSS that represents those that Baron has fielded. The DSS begins with ingest, Q/A, and integration of observational datasets required for forecast model initialization/assimilation or later (offline) evaluation, calibration, and model improvement. Baron ensures that observations are properly quality-assured before being accepted into either a conventional relational database or similar, depending upon the size and type of dataset. Once available, quality-assured observations are then provided to the modeling component(s) of the DSS in real-time.
The forecast modeling system then integrates, typically consisting of an assimilation cycle and a forecast cycle. For example, within the Baron Land Data Assimilation System (BLDAS), operational radar-derived, gauge-corrected QPE and other forcing variables are provided and updated half-hourly. The BLDAS then keeps up with the clock while serving the initial conditions needed to launch forecast model runs. This ensures the forecast model begins with the best possible initial state, which is crucial for hydrological, agricultural, roadway, or other forecast applications. The DSS also provides workstations and displays used by customer staff to interpret forecast information and guidance resulting from the DSS.
Baron offers several options for disseminating threats and warnings, including its widely-used Safety Net application. Typically, the DSS archive provides for observational, model forecast, and forecaster product repositories to fully reproduce, study, and evaluate operational cases. This allows the customer to later improve the performance of the DSS as measured against objective metrics, including categorical, discrete, and areal-categorical measures. Baron also provides an end-to-end customized calibration sub-system that fully interfaces with its hydrological forecast modeling DSS and end-to-end system training and manuals.
Forecast Model Classes and Model Linkages in a DSS Environment
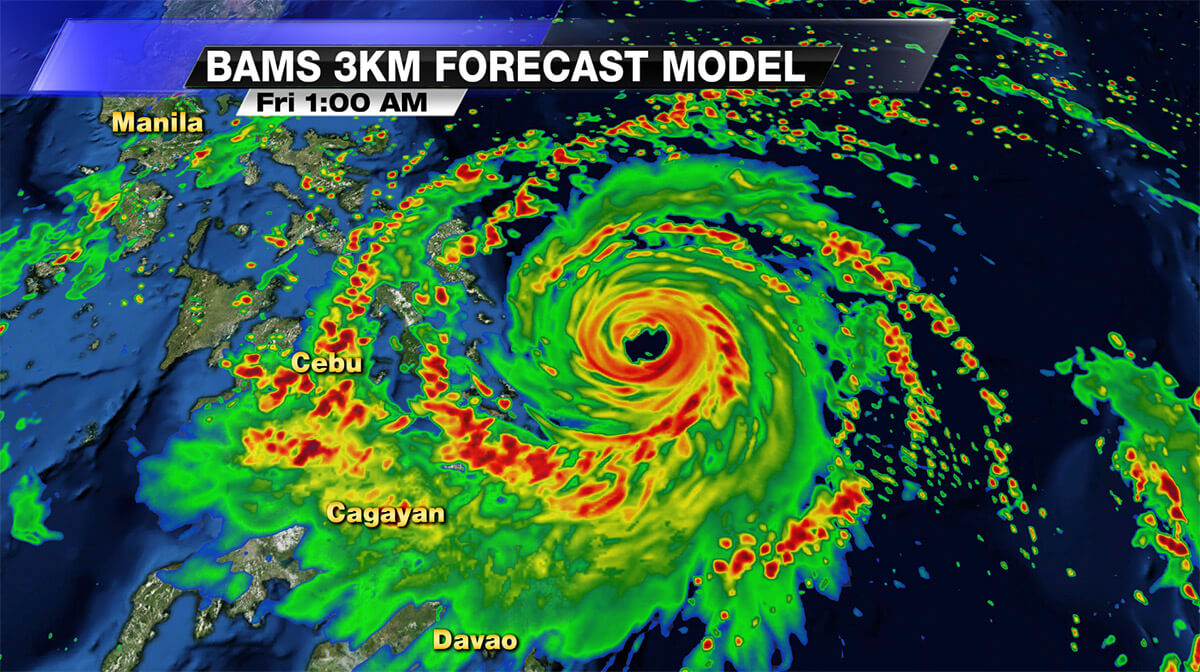
Most DSS forecast applications, including what might be termed “standalone application models (SAMS),” require some form of numerical weather prediction (NWP) model or NWP inputs. Baron’s wealth of experience with the Weather Research and Forecasting (WRF) model (Figure 2), its data-assimilation (DA) systems, and its predecessor MM5 allows it to address many of the current NWP needs that customers may have.
Baron scientists are deeply familiar with the physics, dynamics, and DA schemes within the models, allowing for customizations and parameterization improvements. For example, modifications to the Kain-Fritsch convective scheme (Kain, 2004) reduce spurious tropical cyclone formation, and alterations to LSM, PBL, and microphysics schemes improve land-atmosphere coupling and hydrometeor conservation.
Baron also employs code optimization strategies that improve run times over community-released code versions. This is crucial to getting the most value out of hardware that may also have to be purchased as part of DSS implementation, resulting in significant customer savings or improved workloads compared to community-released model versions. Baron also provides downscaled NCEP GFS deterministic and ensemble-based forecast solutions and can deploy regional-mesoscale models as ensemble systems. This includes implementing NWP models developed within countries or consortia outside the US.
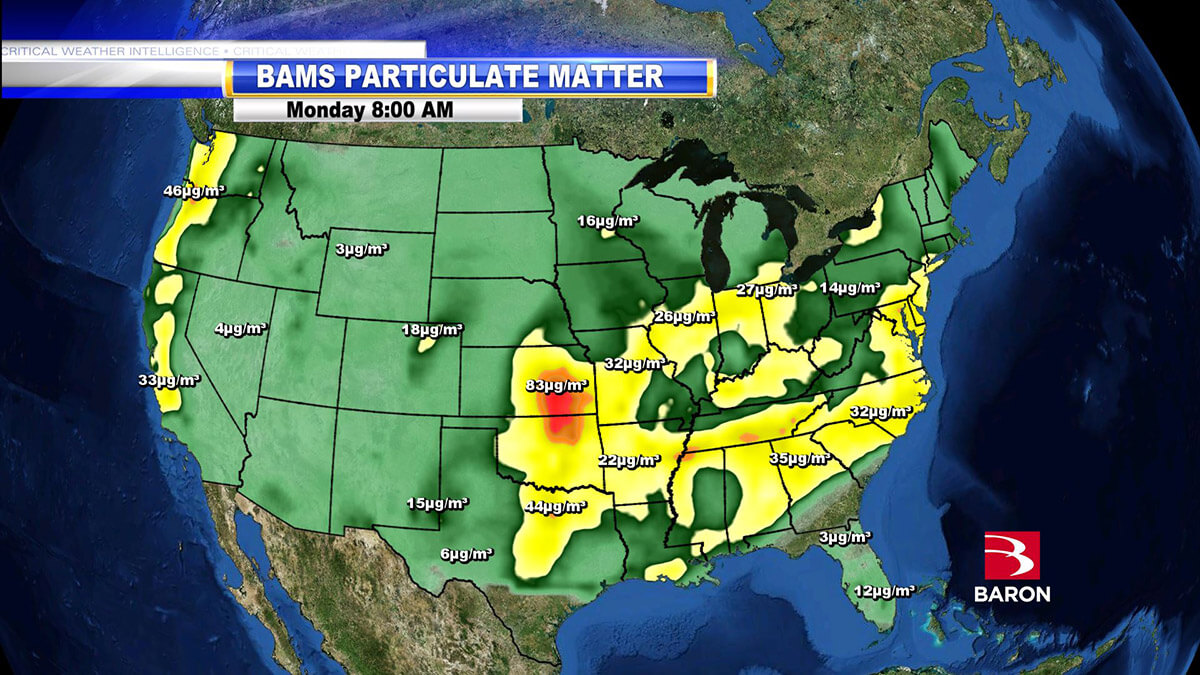
The implemented NWP model feeds and is coupled to other application models, such as air quality, hydrology, or various land-surface-based SAMS within many Baron DSS applications. For example, Baron has provided operational air quality forecast guidance to numerous US customers for over a decade (McHenry et al., 2004; Figure 3). This requires running either a tri-partite “loosely coupled” modeling system (NWP, emissions, and chemical-transport model, such as the US EPA Community Multiscale Air Quality (CMAQ) model) or a more tightly coupled, integrated modeling system such as WRF-Chem.
Furthermore, Baron’s recent development of variational assimilation of NASA satellite (MODIS) aerosol optical depth information into the CMAQ modeling platform (McHenry et al., 2013; Figure 4) allows that model to realize significant improvements in particulate matter forecast skill depending on the quality of the satellite-derived estimates. A principal challenge with high-accuracy forecast AQ-DSSs is the dynamic emissions estimates, which need almost continuous attention to updates. Baron scientists developed the Sparse-Matrix Operator Kernel Emissions (SMOKE, Coats and Houyoux, 1996) processing system for the U.S. Environmental Protection Agency to address this issue, and Baron utilizes a real-time version of that system to provide emissions to its operational air quality models.
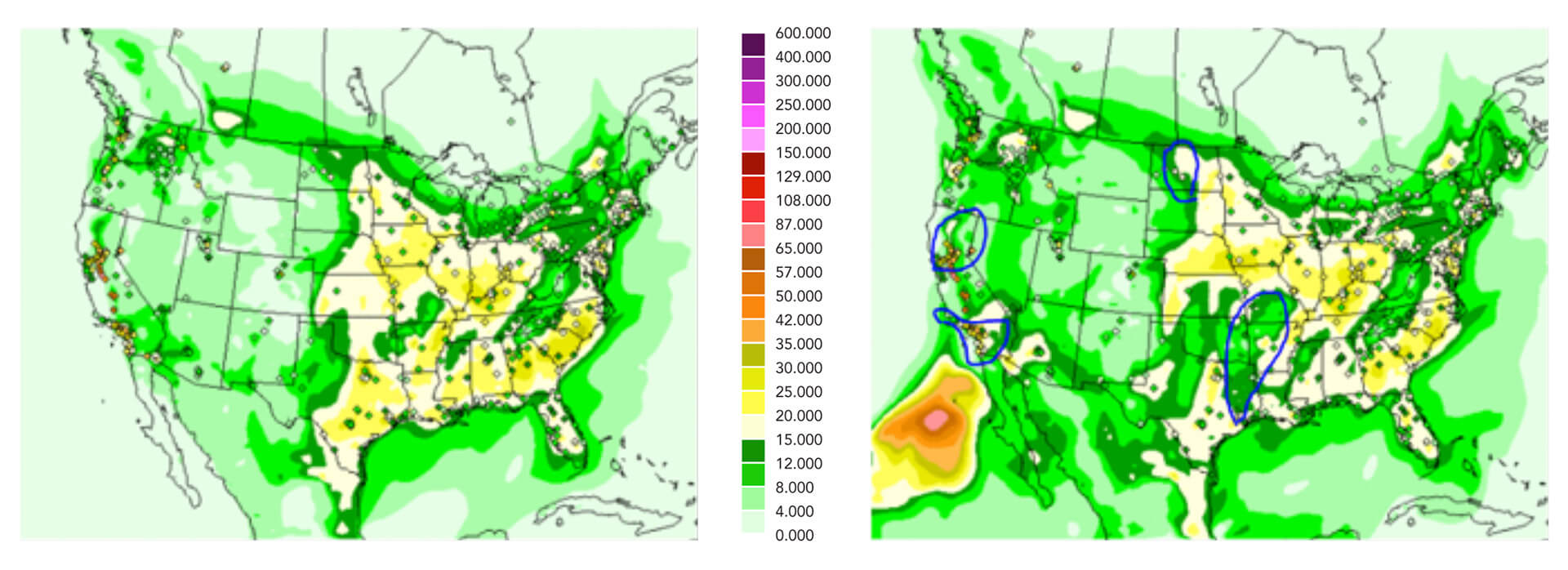
As indicated above, NWPs are also used – in combination with observations – to drive a wide variety of land-surface-based and hydrological modeling systems. In the US, the BLDAS runs natively at 1km spatial resolution across the entire CONUS using input QPE at that same scale. This represents the largest operational implementation of a very-high-resolution LDAS available (McHenry and Olerud, 2015). In addition to streamflow hydrology, assimilation and forecast versions of the BLDAS are used as a basis for agricultural, roadway condition (Figure 5), fire weather (Figure 6), and flash flood threat SAMS.
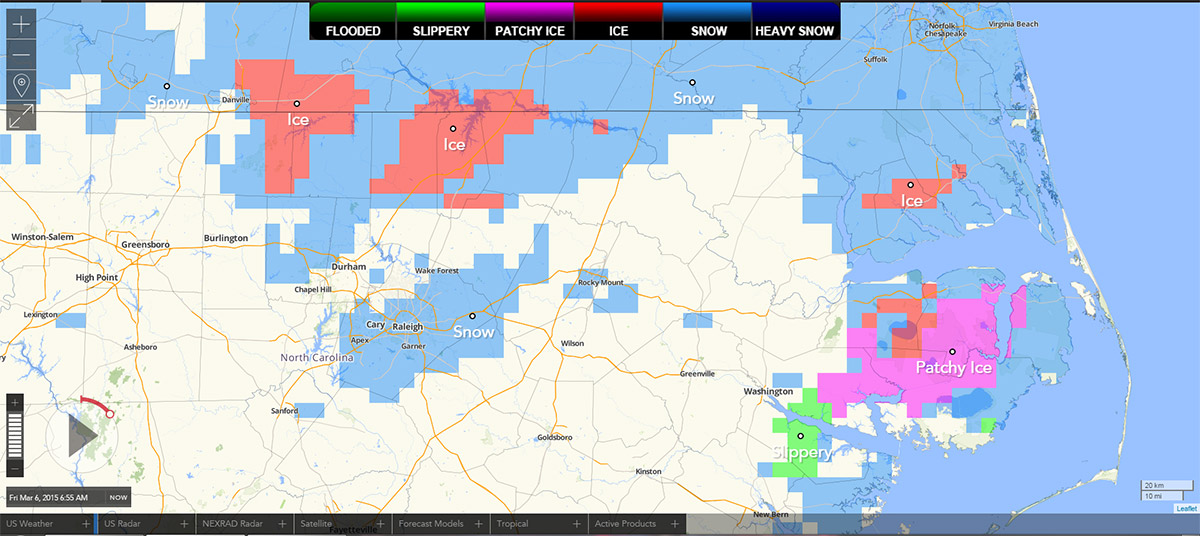
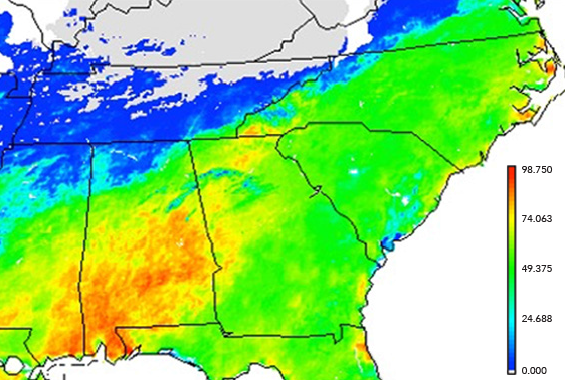
For agricultural applications, BLDAS provides inputs to corn and soybean maturation models, as well as post-maturity dry-down models. Running in ensemble mode, the crop maturation models allow probabilistic interpretation of maturation and optimal harvest forecast dates, permitting farmers to plan for or hedge against either early or late probabilities of crop maturation and harvestability. While corn and soybeans are the primary targeted crops in the US, the Baron database applied within BLDAS permits soil moisture and temperature conditions to be estimated for more than 90 different crop varieties at their growing locations, including both annuals and perennials.
The Baron pavement modeling system also uses inputs from BLDAS and other observational data and models to provide pavement temperature, water content (frozen or not), and driving conditions estimates in real-time. This system has been available through the XM NavWeather service in many OEM car models in the US for several years now. NavWeather provides actionable, hands-off information directly to the driver to result in better anticipation of poor driving conditions ahead.
Providing high-resolution real-time flash flood threat guidance is a challenge, particularly when geographic coverage needs to be complete and products must be rapidly updated. Baron has now deployed a flash flood guidance system called the Rapid Early Flash-flood EXamination (REFLEX) SAM. REFLEX combines local-direct (within grid cell) runoff amounts with runoff routing through a grid-cell to grid-cell stream network that is applicable for up to six hours of upstream runoff that would accumulate at each target grid cell. By running the lag-routed-runoff model for 30 historical years and saving half-hourly outputs, extreme event statistics are captured for each grid cell in the modeling domain, and then used as a benchmark against real-time/forecast accumulated runoff. Recent case studies in the SE US showed that for two events near the Raleigh-Durham area in the state of North Carolina, REFLEX estimated a significant threat potential for flash flooding well ahead of flash flood warnings (Figures 7 and 8).
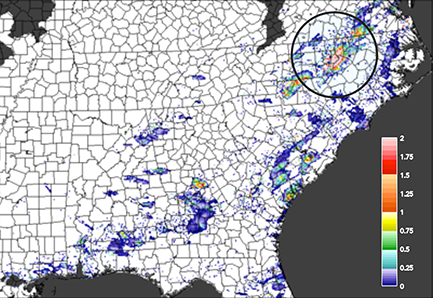
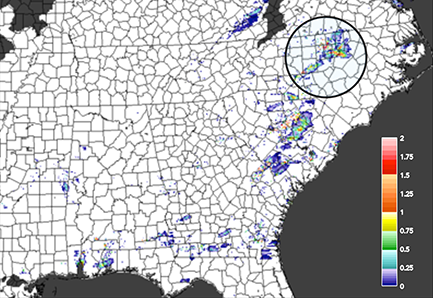
Baron completed installation of the Hydrological Forecast Modeling System (HFMS) for the Romanian Destructive Waters Abatement Program (DESWAT), which has now been operational country-wide for three years. A recent case study published by Matreata et al. (2013) evaluated the Baron explicit streamflow forecast model (designated NOAH-R in that paper) running at 100-meter resolution for a flash-flooding event that occurred in the Calnau River basin on 24 and 25 July, 2011. Peak discharges at the two hydrometric stations in the basin corresponded to return periods of 20-100 years. Spatial and temporal distribution of the intense precipitation event was correctly indicated by the Baron Hydro FutureScan radar extrapolation sub-system while the explicit streamflow model forecast the observed hydrograph quite well (Figure 9). As noted by Matreata et al., other gridded products provided by the Baron system (soil moisture state, overland runoff, subsurface runoff) are “very useful as supplementary and/or support information during the flash flood warning process. They can be especially helpful in better estimation of the severity of the expected flood event.”
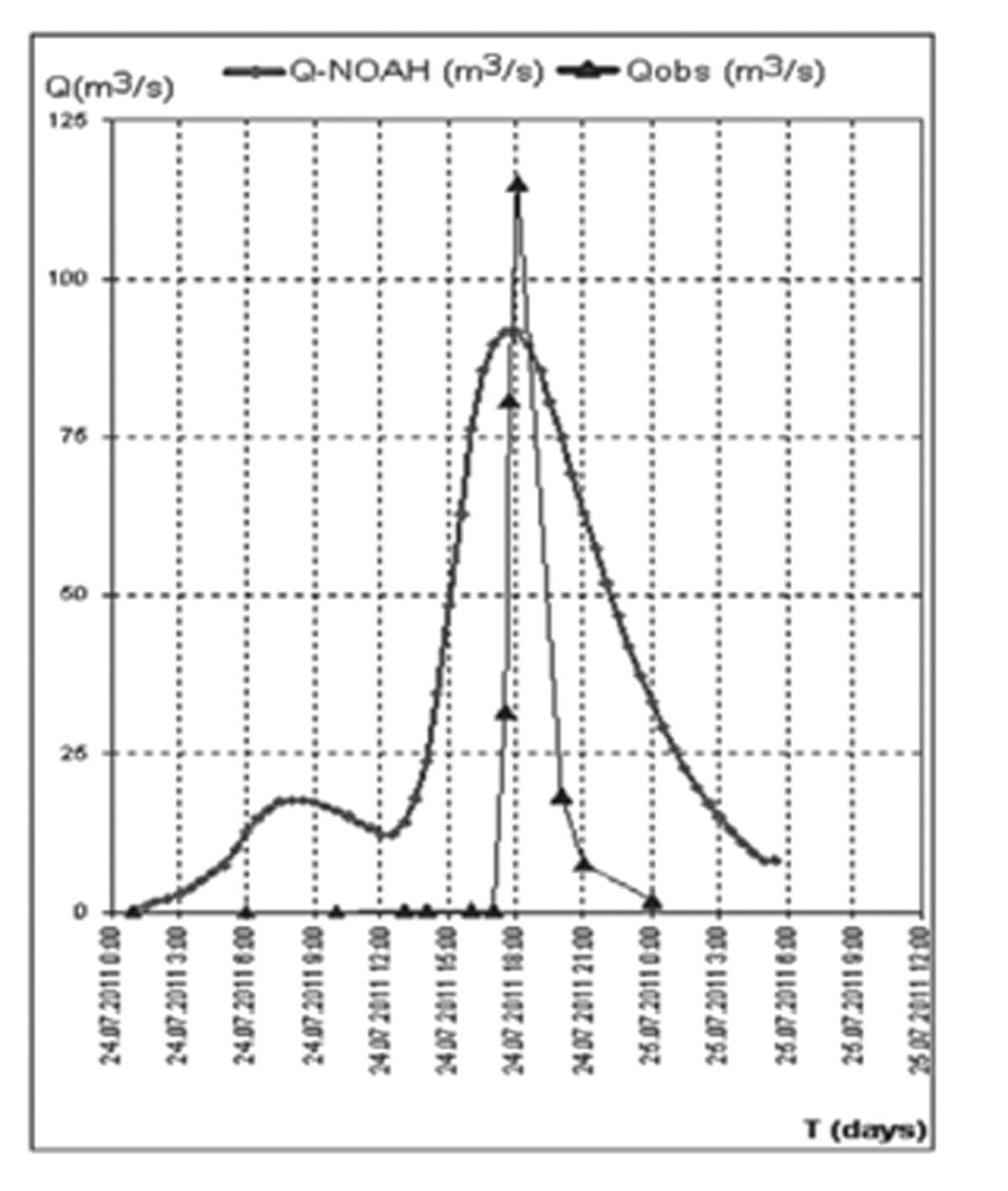
Critical Weather Intelligence
Baron forecast model-based decision support systems are making a significant contribution in providing the critical weather intelligence needed across a broad spectrum of decisions that are weather sensitive. Future articles will detail the various classes of DSS that are available and the advantages they bring to current and potential customers.
References
Coats, C.J. and M.R. Houyoux, 1996: “Fast Emissions Modeling with the Sparse Matrix Operator Kernel Emissions Modeling System,” Presented at “The Emissions Inventory: Key to Planning, Permits, Compliance, and Reporting.” Air and Waste Management Association, New Orleans, LA, September 1996.
Kain, J.S., 2004: The Kain–Fritsch Convective Parameterization: An Update. J. Appl. Meteor., 43, 170–181.
Matreata, S., Baciu, O., Apostu, D., and M. Matreata, 2013: Evaluation of the Romanian flash flood forecasting system – a case study in the Calnau River Basin. Die Bodenkultur 64(3-4), 2013, pp. 67-72.
McHenry, J.N., W.F. Ryan, N.L. Seaman, C.J. Coats Jr., J. Pudykeiwicz, S. Arunachalam, and J.M. Vukovich, 2004: A Real-Time Eulerian Photochemical Model Forecast System: Overview and Initial Ozone Forecast Performance in the NE US corridor. Bull. Amer. Met. Soc. 85, 4, 525-548.
McHenry, J.N. and D.T. Olerud, 2015: Use of a Real-Time High-Resolution CONUS LDAS to initialize land-surface states in operational NWP models: case studies, results and challenges. 29th Conference on Hydrology; 95th Annual Meeting of the American Meteorological Society, Phoenix, AR, 4-8 January, 2015.